Remote Sensing for Large-scale Agricultural Investment Areas
Monitoring agriculture in Ethiopia based on an earth observation time series
Monitoring agriculture in Ethiopia based on an earth observation time series
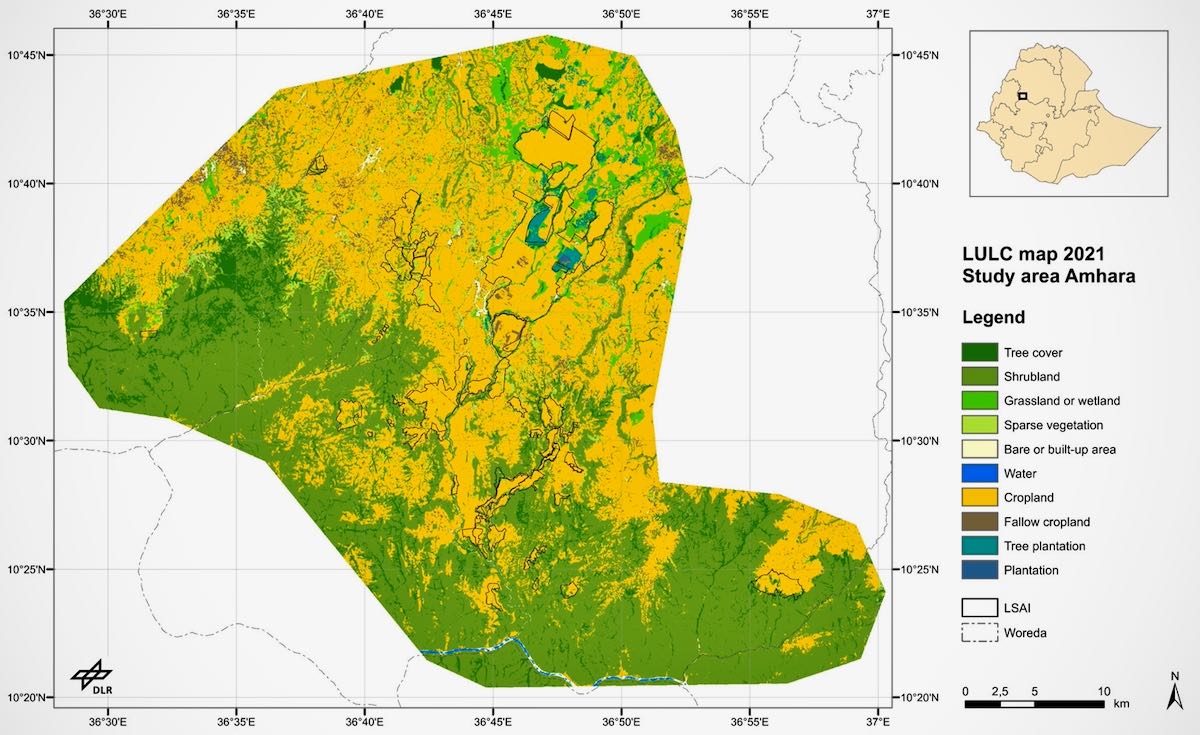
Land use/land cover map 2021, study area Amhara⎮ Photo: © German Aerospace Center (DLR)
Ethiopia, currently grappling with food deficits and food insecurity, has prioritized large-scale agricultural investments to transform its agricultural sector. However, the progress in agricultural development has been limited, highlighting the crucial need for monitoring the implementation and status of land use in these projects.
This study introduces remote sensing methods aimed at supporting and enhancing agricultural monitoring in Ethiopia.
Informative outputs for agricultural investment areas
The developed classification methods have been successfully utilized to generate informative outputs for three study regions in Ethiopia, specifically focusing on large-scale agricultural investment areas in Amhara, Benishangul, and Gambella provinces.
These methods and derived information products are now accessible to GIZ and regional experts, contributing to the development of more efficient and effective monitoring strategies for agricultural investment areas in Ethiopia.
The Remote Sensing for large-scale Agricultural Investment Monitoring in Ethiopia (RS4AIM) project and its subsequent RS4AIM-2 initiative align with ongoing efforts to improve the effectiveness of land management interventions in Ethiopia.
Remote Sensing for large-scale agricultural investment areas in Ethiopia – agricultural monitoring based on Earth observation time-series ⎮ Poster: © German Aerospace Center (DLR)
Methodology
To assess land use for agricultural production and differentiate between crop types within the three study areas, an analysis of earth observation data time series was conducted.
The current land use/land cover was determined by utilizing Sentinel-2 and Sentinel-1 time series data, along with digital elevation model data to identify cropland areas. Subsequently, time-series data from Sentinel-1 and Sentinel-2 were employed to distinguish among 20 different crop types cultivated in these regions.
The process involved supervised classification, using field data on crop types and sample data on land use/land cover, and training and applying random forest classifiers with selected spectral and temporal metrics.
The cloud processing environment of Google Earth Engine was chosen, facilitating the utilization of the classification procedure by regional experts without the need for extensive computational power or software.
Contact
Genanaw Alemu (Genanaw.alemu@giz.de), Rahel Hailu (rahel.hailu@giz.de)